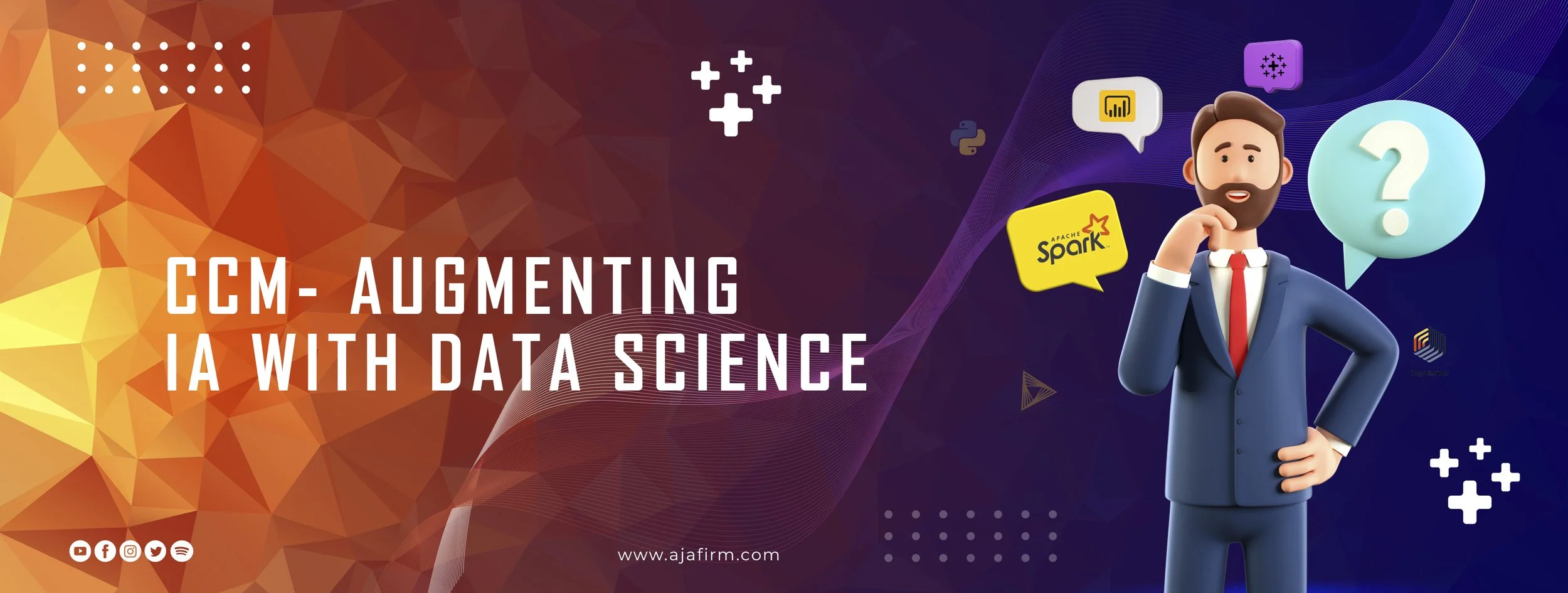
Table of Contents
Potency of internal audit
Who is the new entrant in technology revolution?
Is Data Science a cure for “Data rich, Information poor scenario”?
How data Science augments the purpose of Internal Audit?
Is up skilling the need of the hour?
Why people who can make meaningful insights, make better CEOs?
Takeaways…
A sneak peak of Future
The potency of internal audit
An Internal audit is an independent and unbiased recommendations to boost the efficiency and effectiveness of the business and add value to the organization.
It needs level of power and responsibility for Internal auditors who are reporting to audit committees. The irony is, none like to get audited, yet they are quite aware of the importance of being audited.
The internal audit function of organizations is under pressure to deliver results to meet the assurance demands of stakeholders and protect the organization from emerging threats. Keeping the big picture in perspective, it is difficult given the volume of data and reports, which exist within an organization, a risk-based assessment is the best that could satisfy the job on hand. But how can the constraint of full data review that sampling methods innate limitation, be cured?
Who is new entrant in technology revolution? Who is the new bee? / What is data science?
Data science combines multiple fields, including statistics, scientific methods, artificial intelligence (AI), and data analysis, to extract value from data. The data used for analysis can come from many different sources and presented in various formats. Internal audit departments rely heavily on mining data in the overall audit process, internal audit data analytics is becoming increasingly necessary.
The exponential growth of data increases the complexity of the audit process. By incorporating data analysis into the internal audit function, Chief Audit Executives and internal audit teams can wade through the overwhelming amounts of data. They’re able to extract more effectively the information they need to produce more robust and in-depth reporting.
Sophomore / Few Data analytic tools
Is Data Science a cure for “Data rich, Information poor scenario”?
Internal reporting has crossed into the era of big data, and this is leading to information overload across the corporate landscape. There is the lack of skill required to make meaningful information from raw data, so that’s when technology chips in as a saviour. Data analytics for internal audit can help you spot and understand the risks by quickly reviewing large quantities of data. Data analytics can provide a more systematic, complete review of business processes that make it easy to see whether findings from different departments align. For example, an internal audit team might use data analytics to review financial data such as transaction logs to see if there are any anomalies.
How data Science augments the purpose of Internal Audit?
The first step of using data science involves gathering raw and unstructured data. Next step would be data cleansing by taking the raw data and placing it in a form that can be used. Then follows the Data Mining and Data modelling using tools like KNIME to prepare the data and examine its patterns, ranges, and biases to make predictive analysis on data. Some level of programming like Python are used as it supports multiple libraries for data science and ML. Finally, data reporting, BI, Data visualisation to prepare the analyses in easily readable forms such as charts, graphs, and reports.
Is Upskilling the need of the hour?
Financial audit is focused on the detection of potentially material issues in recordings of transactions, and at times, corrections. In other words, losses detected in a financial audit have already occurred, while the results of internal audit’s work highlight gaps in compliance, quality, and other areas. To identify these gaps, internal audit operations require the tracking of outcomes in each risk area that the organization’s programs are exposed to. This tracking requires contextualization and fast results, rather than an annual summary report to the audit committee. There is a tremendous amount of upskilling which is required because of the nature of risk that is spiralling upwards. There is also need of hour for individuals who can use data. What traditional internal audit was performing was a mere post-mortem of the trail/transaction, but is prevention or uncovering the anomalies closer to its occurrence, not a better solution? Data science accelerates to reach our true calling!
Why people who can make meaningful insights, make better CEOs?
Businesses are becoming increasingly data driven and CEOs who are powerhouses in leveraging data to optimise business operations will be crucial to achieving the goals of the business.
Whilst they may not dig into the data the entire day, they function in highly correlated capacities to a data analyst, but at a more senior and strategic level. A data driven CEO looks for patterns in data before forming any conclusions. With the ever-increasing importance of technology and Data Analytics in modern business processes, business executives and particularly CEOs must have an excellent grasp of how the business can leverage these technologies and the large amounts of data generated to gain advantages over competitors and directly increase revenues or decrease costs.
Interestingly there are couple of CEOs who started as Data Scientists, Sebastian Thrun, executive chairman of Udacity, Thomas Thurston, a venture capitalist, a CTO of Growth Science. Sri Satish Ambati co-founder and CEO at H2O.ai. Michael Berthold, President, and founder at KNIME, an open and extensible data science platform and Peter Lee, CEO of RapidMiner Inc.
Takeaways…
A sneak peak of Future/ Roadblocks or Deterrent Vs Reforming the outlook of IA
Because data quality plays a fundamental role in the success of an internal audit data analytics program, a specialized skill set for understanding and managing data analytics capabilities is necessary. The inability to apply data science to internal audit methodology is a common reason many organizations have not fully implemented data analytics functions into their internal audit planning.
According to a Deloitte survey, most of the large companies are not mature when it comes to business analytics and 62% still rely on spreadsheets. Even though there is a been an increase in the number of organizations that have adopted advanced analytics in their business processes, most are just data analytics dabblers and only a few have embraced data literacy, adopted analytics, AI, and automation, and systematically transformed the way work is done across the enterprise.
The lag in adoption of natural language processing and machine learning in internal audit, relative to other fields such as law and accounting, could be explained by institutional inertia, a lack of training datasets, the reimbursement model for consultants, the requirement to understand documents in multiple languages, and differing standards for reporting. These various factors holding back the field are now shifting, leading to a major opportunity for audit automation with machine learning.
But the road map to the desired destination will materialise when Data Science permeate company’s culture starting from top to bottom.